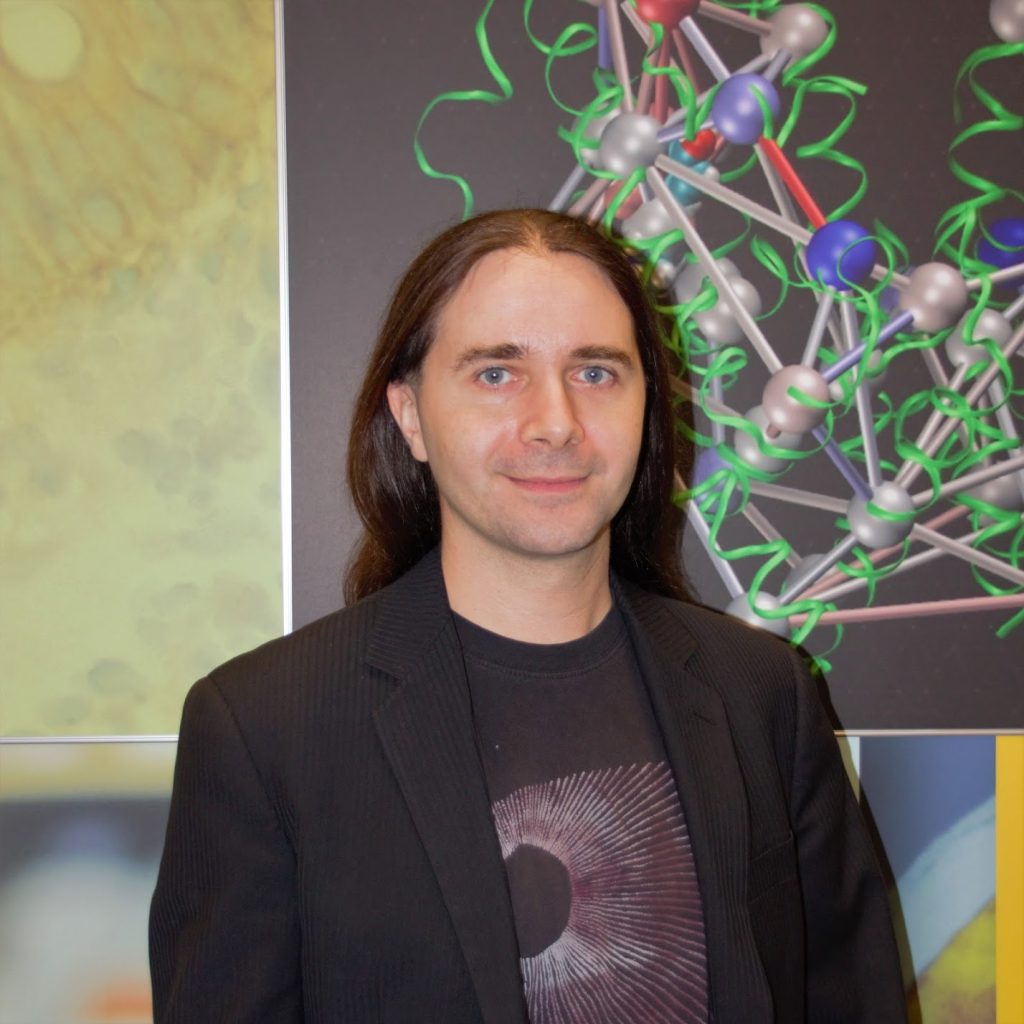
[email protected]
Associate Professor, Department of Chemistry, University of Tennessee
Research focus: Quantum Theory and Simulations, e.g. AI, high performance computing, topology, algorithmic development, correlated systems.
Website https://vogiatzis.utk.edu
Follow on https://twitter.com/VogLab (Twitter)
Bio
Konstantinos “Kostas” Vogiatzis completed his bachelor’s degree in chemistry at the University of Athens, Greece, in 2006. In 2008, he obtained his MSc in Applied Molecular Spectroscopy from the University of Crete, Greece, and he received his Ph.D. in 2012 from the Karlsruhe Institute of Technology, Germany. After an eight-month post-doctoral appointment at the Institute of Nanotechnology at the Karlsruhe Institute of Technology, he moved in 2014 at the University of Minnesota, where he performed post-doctoral research at the group of Prof. Laura Gagliardi. In 2016, Dr. Kostas Vogiatzis joined the University of Tennessee, Knoxville, as an Assistant Professor of theoretical and computational chemistry and in 2021 he was early tenured and promoted to Associate Professor. His research group is currently developing new computational methods based on electronic structure theory and machine learning for the theoretical examination of reactivity, catalysis and separation processes. Kostas is the recipient of the 2020 Ffrancon Williams Endowed Faculty Award in Chemistry, the ACS OpenEye Outstanding Junior Faculty Award for Spring 2021, and the 2021 NSF CAREER award.
Research Description
Our group develops algorithms that interface quantum chemistry with artificial intelligence and quantum information for solving problems related to molecular systems.
Recent research
Data-driven quantum chemistry:
1. J. Townsend, K. D. Vogiatzis, Data-Driven Acceleration of the Coupled-Cluster Singles and Doubles Iterative Solver, J. Phys. Chem. Lett., 2019, 10, 4129. (http://10.1021/acs.jpclett.9b01442)
2. J. Townsend, K. D. Vogiatzis, Transferable MP2-Based Machine Learning for Accurate Coupled-Cluster Energies, J. Chem. Theory Comput., 2020, 16, 7453. (http://10.1021/acs.jctc.0c00927)
3. G. M. Jones, P. D. V. S. Pathirage, K. D. Vogiatzis, Data-driven Acceleration of Coupled-Cluster Theory and Perturbation Theory Methods, in: “Quantum Chemistry in the Age of Machine Learning”, 2022, Editor: Pavlo Dral, Elsevier, pp. 509-529. (https://www.elsevier.com/books/quantum-chemistry-in-the-age-of-machine-learning/dral/978-0-323-90049-2)
Research Image
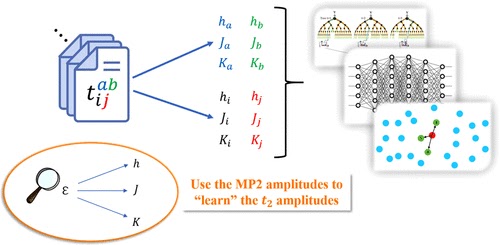